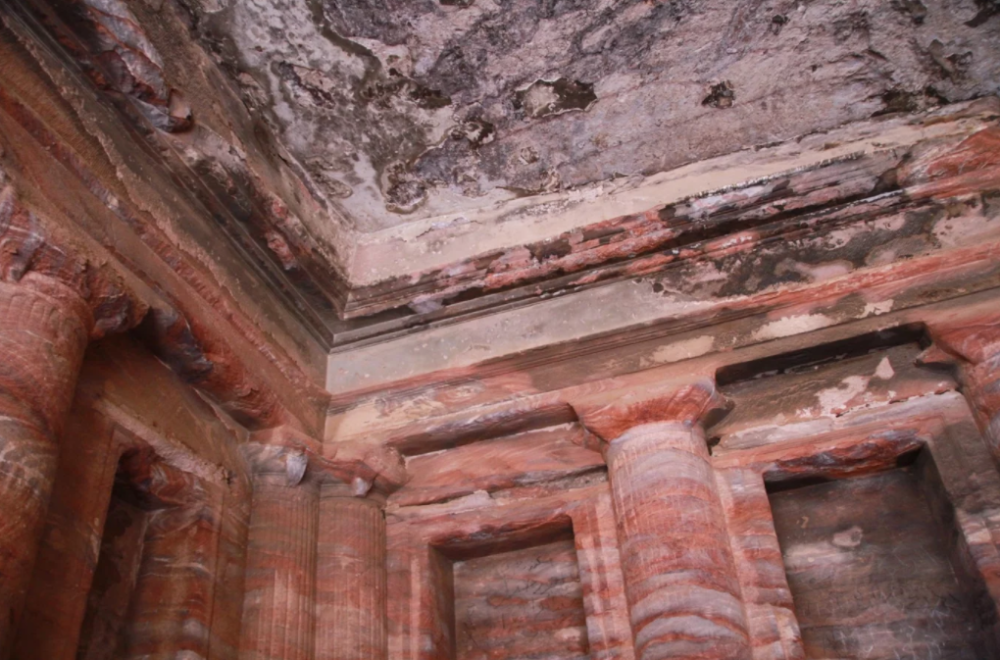
Decisions we make, in business and in life, are a function of the way the evidence we get, the inferences we make from that evidence, the background beliefs that shape our thinking and the way we connect all these inputs to our objectives. Every once in a while, a bad decision bears the fingerprints of just one element gone wrong – but usually our worst decisions are the product of many pathologies that reinforce one another.
The entrepreneur believes the “hockey stick” acceleration of revenues is almost here, so he puts his head down and focuses on pushing the company just a little bit harder down the path he sees. He doesn’t spend much time considering alternative frames, and his board inconclusively debates various metrics, some of which fall far short of expectations, others of which point to strong reason to believe in the current strategy. The VCs are busy, and have sent the message that they want their founders to swing for the fences. The founder doesn’t want to distract his team from operations. Money starts running out, and the company, with too little time for changing course even to feel like a reasonable option, runs into a wall at sixty miles an hour. In this respect, the downfall of the visionary and the downfall of the complacent bureaucrat at the top appear remarkably similar. Each is trapped in a system of thinking too stable to meet the demands of his shifting circumstances. Each fails to revise his thinking, even when his work life depends on it.
What we believe today is largely a product of what we believed yesterday. The act of changing beliefs is disorienting, exhausting and, if done indiscriminately, likely to be wrong. Good decision-making is in significant part the art of figuring out which of our beliefs we change, when, and by how much.
The cornerstone idea in this field is Bayesian reasoning. Bayes’ Theorem, named after an 18th century Presbyterian minister and statistician, is known as the “Pythagorean theorem of probability.” The easiest way to understand Bayes’ Theorem is as a way of thinking about how much a signal tells us to revise our beliefs about a proposition that matters to us. For instance, we might care about whether someone who works for us is going to fail to complete a project on time – let’s call that the proposition (“P”). We might see that he just missed his first milestone – let’s call that the signal (“S”). Bayes’ Theorem states the following:

Let’s apply this to three realistic scenarios:
- “The rookie project manager.” In this first scenario, the supervising executive never thought there a very good probability the rookie project manager could deliver on time – perhaps she thought that a miss (P) was 70% likely. Supposing that it turns out the rookie misses the final deadline, the sponsor thinks it’s 50% likely (S, if P) that the rookie would miss the first milestone along the way – plenty of opportunities to hit the first milestone but still fall behind later. If the rookie does end up succeeding in delivering on time, it’s only 25% likely (S, if not P) that she missed the first milestone – while it is possible that the rookie would make a mistake and recover, her chances of “coming from behind” aren’t great, given her inexperience and the expectation that there will be many further challenges along the way.
- “The wizard of the last minute.” In the second scenario, the supervising executive has seen the project manager pull rabbits out of hats, delivering projects even when it seemed all hope had been lost. She knows the project manager is a procrastinator, but impressively skilled and committed to delivering the right result at the end. In this scenario, perhaps the executive believes up front that the project is only 10% likely (P) not to be delivered on time. Assuming the project does come in on time, it’s still 70% likely the procrastinator is behind at the first milestone (S, if not P). In the case, that the wizard isn’t able to pull out the project at the last minute, it is 80% likely he was behind at the first milestone (S, if P).
- “The meticulous project manager who hates to give a bad report.” In the third scenario, the project manager has a proven track record, and the sponsor thinks it’s only 10% likely that he misses the final deadline (P) – the same odds as the “wizard” in scenario two. In this scenario, however, the project manager is extremely careful not to bring bad news. He’ll hit the first milestone if there’s any way he can. The sponsor therefore believes that the chances he’d report a miss at the first milestone and that the project would ultimately succeed are low (S, if not P) – perhaps 5%. The only way he’d report a miss is if something were seriously, seriously wrong – so wrong it probably wouldn’t be recovered from. If this project manager fails, the chance that this would be evident at the first milestone (S, if P) is 30%. If something does go wrong, it might well go wrong later in the project, in which case nothing would be evident at milestone one.
Plugging these numbers into the Bayes’ formula yields the following:
Project Manager | Sponsor’s view of likelihood to miss – Day 1 | Sponsor’s view of likelihood to miss, after the missed milestone |
Rookie (Scenario 1) | 70% | 82% |
Wizard of Last Minute (Scenario 2) | 10% | 11% |
Meticulous, Hates to Give Bad Report (Scenario 3) | 10% | 40% |
The results reflect what is intuitive in each situation. The sponsor was pessimistic about the rookie from the beginning but wanted to give him a shot – hopefully the consequences of missing the deadline aren’t too bad. When the rookie misses his first deadline, there’s a pretty high likelihood that the signal is confirming the pessimistic prior belief, but the sponsoring executive can’t be sure. There’s a reasonable chance that this signal is a false indicator, and that the rookie will get his sea legs and find a way to bring the project home on time. With the procrastinating wizard, the missed first milestone tells us almost nothing and leads to almost no revision in the odds of failure. The explanation is probably just the procrastination the sponsor expects, nothing the wizard can’t recover from. She’s seen this movie before, and it ends well. With the meticulous project manager, we learn much more from the signal. When we see the missed milestone, the probability of a bad outcome surges. The signal tells the sponsor that her initial view (“this project manager has a great track record, he’s 90% likely to deliver”) is fairly likely to be wrong. It’s not very likely that the missed milestone is an accident, just a bump along the road to a successful outcome, so the sponsor gives the negative data much greater weight than she does with the wizard.
Usually when statisticians present examples of Bayesian reasoning, their main point is how much better this tool helps one to reason about probabilities. For instance, if a young woman gets a positive indicator in a first test for breast cancer, she shouldn’t panic. The baseline probability of having cancer is low, and the test frequently generates a false positive. So the odds of a false signal are much higher than the odds of the woman who has just received the concerning test actually having cancer. All this is both true and important. My main point here, however, is for the flawlessly Bayesian executive, the picture in her head (what statisticians call her “prior beliefs” or “priors”) shapes what she makes of the evidence at hand. If I think you’re a rookie skiing a hard slope, I’ll think one thing when you slip; if I think you’re a procrastinator operating well within the envelope of your skills, I’ll think something quite different.
Most real-world situations executives find themselves in resemble my example here more than the breast cancer example, where there are objective, clinically validated baselines that can shape our priors if we do a little research and consult a good doctor. Executives have pictures in their heads – priors – about an awful lot of things that connect to any given situation they face. In this example, for instance, the executive has priors about how hard the project is, relative to others; when in the project the greatest difficulties are likely to emerge; what the odds of lateness are for projects in general; how strong each project manager is; how well his or her skills fit this particular problem; how likely he or she is to report bad news; how quickly he or she will get back on his feet if he experiences a reversal; and so on.
Some executives aren’t especially sensitive observers, and these executives – who tend not to be especially good ones – are rarely surprised by anything they see. The best executives have a visualization of the range of ways they expect to see things unfold, so they often find themselves at least a little bit surprised (e.g., “I thought the rookie might miss the first milestone, but I didn’t expect he’d miss and come in with such a buttoned-up plan for getting back on track… he might be a much stronger player than I’d expected”). When we’re surprised – whether very surprised, or just a little bit surprised – we face the question of which of the our many relevant beliefs we should revise.
This question of what to revise is multi-faceted, and it can’t be reduced to a formula. (One could characterize real-life situations as a multitude of different Bayesian calculations all at once, which in a sense they are, but we can’t actually do those calculations all at once.) A valuable lens to bring to complex decision-making is to consider the question of when to revise beliefs “low down” on one’s personal hierarchy of beliefs – not especially tightly held, either intellectually or emotionally – and when to entertain revising beliefs “high up” on one’s personal hierarchy, which are often fused very deeply into one’s picture of the world at both an intellectual and an emotional level.
Some of the greatest moments in business history revolve around leaders making correct revisions to beliefs very high in their personal hierarchies. For instance, in an earlier piece I quote Andrew Grove’s book Only the Paranoid Survive:
I remember a time in the middle of 1985, after this aimless wandering had been going on for almost a year. I was in my office with Intel’s chairman and CEO, Gordon Moore, and we were discussing our quandary. Our mood was downbeat. I looked out the window at the Ferris Wheel of the Great America amusement park revolving in the distance, then I turned back to Gordon and asked, “If we got kicked out and the board brought in a new CEO, what do you think he would do?” Gordon answered without hesitation, “He would get us out of memories.” I stared at him, numb, then said, “Why don’t you and I walk out the door, come back in and do it ourselves?”
Up until that time, the idea that the memory chip was Intel’s core business was right up near the top of their hierarchy of beliefs. Moore and Grove naturally didn’t throw out that belief after the first negative data point crossed their field of vision. Most executives would never have thrown out that belief. That Grove and Moore did made all the difference.
It’s easy to read today’s management books and come to the conclusion that in a “VUCA” world (volatile, uncertain, complex, ambiguous), one should throw out beliefs high up on one’s hierarchy with abandon. We experience paranoid people as crazy because they throw out beliefs that most of us hold very high up in our hierarchies – e.g., that people are almost never out to get us unless we’ve done them what they perceive to be some terrible wrong, that when we imagine a voice we’re probably just imagining. Business leaders who revise “high up” beliefs frequently are at great danger of swinging wildly – which doesn’t prove whether or not swinging wildly might just be the right appropriate thing to do.
There isn’t one answer here, but I believe there are certain principles to steer by, such as:
- When evaluating a situation, separate out three levels: the individual data points coming out of the situation, inferences that draw on those data points as evidence, and the overall conclusions that build on a range of data inferences and background beliefs
- Build disciplines and skills for navigating “levels in a conversation” so that people can have clear debates about one thing at a time (e.g., in a hiring decision, is it a good inference that X outcome in a past job is an indicator of Y attributes that might be relevant in this new job) versus jumping levels indiscriminately (e.g., “he’s done it before” to “in my interview, he seemed poorly prepared” to “I think we should favor internal candidates for a job like this”)
- Make Bayesian inferences explicit – with or without the algebra – so that people can see the rationality of conclusions others are coming to, even when they are divergent… or so that they can critique mistakes in reasoning
- Entertain what it would look like to revise beliefs low down and high up on your personal hierarchy, as well as on your institution’s collective hierarchy. Avoid revising the high-up beliefs easily, but don’t let them escape scrutiny
Importantly, these principles are far more powerful when they are internalized not just by people but by groups – especially groups with high cognitive diversity (e.g., different priors based on experience, different styles of revising beliefs).
In many corporate cultures, there’s a temptation to ritualize activities like obtaining and interpreting market research. This leads naturally to the double danger of under-revising beliefs (e.g., holding too tightly to assumptions, and interpreting new data through the lens of those assumptions) and over-revising beliefs (e.g., treating market research as “objective” and failing to consider issues like how likely it might be for research to generate false negatives to certain kinds of hypotheses). It’s much more difficult to live in the fluid, constantly self-critical gray area that the principles above imply.
This, however, is what those who best navigate business and life actually do. They may or may not know Bayes’ Theorem, may or may not know the odds of things with any precision. But they’re constantly surprised in small ways by what they observe. They’re constantly generating a wider range of inferences one might make from the same data that others see. When they see something amiss, they don’t revise their views too fast, but they do turn up the dial on their level of effort to find out precisely the things that they’d be most uncomfortable to learn. They experience more tensions among their own beliefs, and are more inclined to resolve those tensions differently in one situation than in the next, rather than to default to a single rule of thumb. They let the world teach them, but recognize how hard it is to know, at any given moment, precisely what it is that they should learn.